AI Ethics from an Assurance Perspective
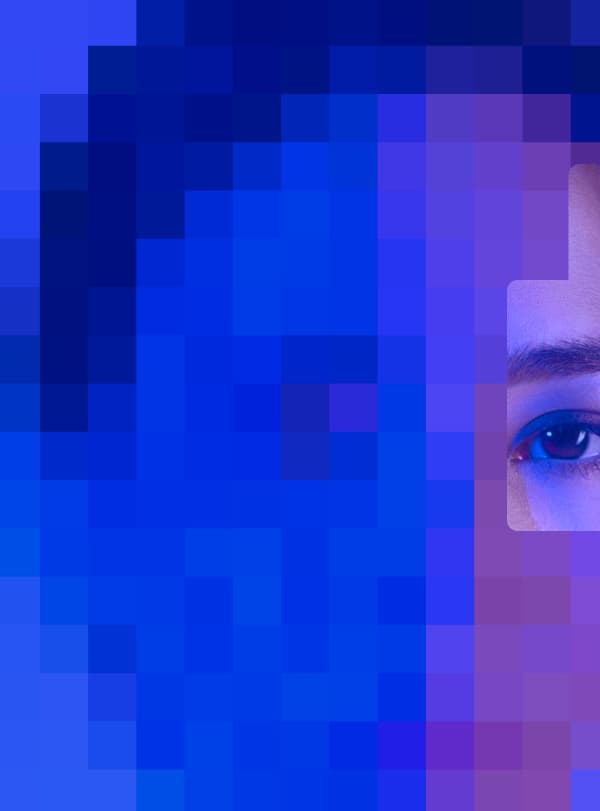
Considering ethical factors is an essential component of good AI Assurance. Some aspects are mandatory compliance requirements, while others are simply best practice.
We highlight key ethical considerations, so our customers can understand and address concerns at each stage, from ideation through development, deployment and monitoring. There’s an Assurance mindset that can be employed to ensure your use of AI is ethical and technical approaches that can be employed to mitigate specific ethical risks.
AI Alignment: Tests that score AI for robustness and resilience are just as important as accuracy metrics. These tests can be an important indicator for proactively understanding whether AI could breach ethical principles.
Diverse, representative Datasets: Attacks can reveal which features are underrepresented and inform which data augmentations are needed to represent various demographics or features, to prevent system biases. This is particularly true in classification systems.
Transparency in Algorithms: Model cards make the system more understandable to users; and an understanding of a system’s weakness clarify scenarios/contexts where an AI model is likely to make good or bad decision/actions.
Regular Audits: Post-deployment, by logging and monitoring AI inputs, outputs and uses, it becomes possible to identify and rectify issues.
Ethical LLM Guardrails: Technical approaches to ensure an AI chat interface retrieves and presents information in an ethically desirable way, filtering out harmful content.
Decision Maker Clarity: Using a tool like Advai Insight bridges comprehension gaps between technicians and decision makers so ethical risks are understood. Two-way communication is vital - ethical objectives need to be communicated and technical assurance against those objectives must be communicataed.
Ensure key legal stakeholder alignment - such as involvement of key compliance personnel - around AI system development and use. Bring legal teams in early stages because some ideas should be stopped before they begin!
Regulations such as:
The AI Act is a European legislative framework seeking to balance AI innovation with ethical considerations and the protection of fundamental rights. The AI Act takes a ‘fundamental rights approach’ by focusing on the impact of the use of AI, versus regulating any specific technology. The legal reasoning for doing this is to create a set of standards that will stand the test of time. European parliament president, Roberta Metsola, refers to it as “legislation that will no doubt be setting the global standard for years to come.”
International organisations should install governance procedures that meet the strictest global standards. For many UK businesses buying, selling or developing AI-enabled services, The AI Act is undoubtedly the clear standard to meet.
The AI Act will be published in the Official Journal of the EU by Mid 2024 and will enter into force 21 days after publication. By Early 2025, the bans on Prohibited AI will apply.
Read our blogs on the topic:
Article 22 of the General Data Protection Regulation (GDPR) states that when a solely automated decision is made, resulting in a legal or similarly significant event, individuals have the right to not be subjected to it.
Further, that the handling of personal data within the EU focuses on principles including lawfulness, fairness, transparency, data minimisation, and accountability.
CCPA
Similar to and possibly inspired by the EU's GDPR regulation, the California Consumer Privacy Act (CCPA) of 2018 extends privacy rights and consumer protection for residents of California, in the United States. Similar to GDPR's emphasis on principles like lawfulness and transparency, the CCPA focuses on safeguarding Californians from unauthorised sales or disclosures of their personal information.
It's interesting to speculate that The AI Act will similarly inspire future AI-specific regulation around the world.
Provides a legal framework to protect against discrimination, ensuring services and employment are fair and inclusive.
These laws protect individuals' fundamental rights and freedoms, impacting how AI interacts with personal data and privacy, or how a systems use might impact somebody’s livelihood.
We’re a long way off fully dependable AI systems. Rigorous failure point analysis determines the boundaries for their safe use and shows organisations where human oversight must remain. Ensure that clear divisions of responsibility are based on respective capabilities. Continuous monitoring will be necessary because the line dividing human and AI capabilities will shift and your organisation must be quick to react to this changing dynamic. This will also enable you to re-train humans and repurpose AI systems.
Continuous assessment of AI systems to understand how users are interacting with AI, to identify and mitigate potential risks of bias, misuse, and unintended consequences. It's an active research field and something Advai are involved with directly for our clients. Technical governance isn't always enough, sometimes you need to ensure the humans involved using the system appropriately. There is a knife-edge to walk that balances trustworthy systems with user over-reliance. On the one hand, organisations should strive for transparent, accountable, dependable and fair systems. On the other hand, organisations must be wary of their users becoming overly dependent on these systems and placing too much trust in the technology.
Discussions and preparedness for AI’s future potential and how this may be accompanied by new ethical concerns. Although current AI technologies may not yet be advanced enough to integrate into certain workflows, rapid progress in the field suggests they soon will be. AI applications once thought to be decades away unfolded within years. Therefore, organisations should proactively consider and prepare for the long-term ethical risks of AI, such as laying down best data management practices. Strategic preparation will ensure you are well placed to mitigate future ethical challenges and enable you to take advantage of new AI opportunities fast.
There are varying opinions and contexts of AI ethics. Enabling clear stakeholder communication, and closely monitoring how internal or external users are using your AI systems, are necessary to incorporate diverse perspectives.
Effective cyber security and adversarial resistance is also an ethical priority, especially in the case that your organisation handles sensitive user data. Strengthening your data protection measures and identifying your AI system vulnerabilities are both vital for respecting and protecting your user privacy. Adversarial techniques exploit system weaknesses, potentially leading to data exposure and privacy breaches. This can occur through methods like model inversion attacks, which reconstruct sensitive information, or due to vulnerabilities in encryption practices and unintended data disclosures via API leaks.
AI can be an energy intensive process and in many cases it’s unclear whether the incorporation of incremental training data will improve performance. There may also be ways of reducing the carbon footprint of AI training/re-training by removing redundant processes. Understanding the tools that are available, such as Advai’s performance predictor model, can help you deploy AI more sustainably.